“With 49 properties any object can be characterized”
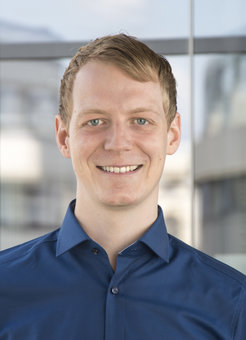
Mr. Hebart, what exactly is your research about?
We would like to understand the principles by which we can recognize an object and interact with it in a meaningful way. For example, how can I recognize the glass in front of me and drink from it? That sounds easy at first. But it is an amazing ability when you consider that the environment around us is constantly changing and we keep receiving new sensory impressions of objects that we have never experienced before. Behind the scenes, if you like, a complex sequence of perceptual and decision-making processes takes place, which allow us to recognise and classify the properties that make an object what it is and that distinguish it from other objects. The findings from our research mainly serve to better understand these processes in the human brain. But they might also help us improve artificial intelligence.
In what way?
Take the example of self-driving cars. Until recently, the main focus of artificial intelligence research has been largely to yield the best possible prediction. For most real-world applications, it is irrelevant how exactly objects are represented by artificial intelligence and how the problem of object recognition is solved as long as they are correctly categorized. However, recent studies have shown that this can lead to problems in situations that artificial intelligence does not know so well. For example, for a self-driving car, it is very important to recognize that a ball on the street means a child could be nearby. Nevertheless, certainly very few images from which these algorithms have learned to drive will contain balls on the street. If you better understand the similarities and differences in the representation of objects in humans and artificial intelligence, this can help making these algorithms better.
How do you want to investigate the recognition of objects?
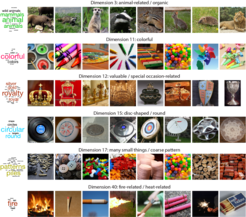
In order to better understand objects, we first had to solve a general problem: What are the objects that exist around us, and which ones should we use to capture this diversity? First, we collected about 7,000 terms for different things. We then distilled them to a set of words that are really used as labels in everyday naming. A “carp” was thrown out because the test participants called it “fish”. We found that with around 2,000 names, i.e. categories, the world can be described quite well. The next challenge was: How do we find pictures of these objects that we can show to our test participants? We browsed image search engines on the Internet and collected about 12 to 15 images from each category. In total, we ended up with around 26,000 files. We cropped all images to the same format and put them together in a large database of images of all objects, which is now also freely accessible for other researchers. Based on this, we are conducting various studies, from behavioral experiments to measuring activity patterns in the brain in response to the images. We support this approach with computational models from artificial intelligence research such as deep neural networks, which, simply said, consist of a large number of artificial neurons that are arranged in several layers. These models are inspired by the brain and are currently the best models we have of visual processing.
What have you found out so far?
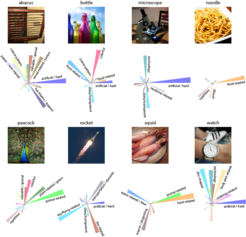
We have just submitted the results of a large behavioral study explaining which properties we would most likely use to characterize the approximately 2000 objects. Ultimately, around 50 so-called core dimensions have emerged, on the basis of which we can recognize all objects and group them into categories, forming the basis for the so-called mental representation of these objects. For example, most people agree that an octopus and a shrimp are more similar to each other than they are to a car. They share more core dimensions with each other - they are living things, you can eat them, they have a strong connection to water, and they are rather slippery. With only 49 dimensions, each object can be characterized very well, so well that we can hardly explain it better with our data. For me, this is really exciting.
We started a study a few months ago in which we wanted to figure out whether our 26,000 objects are reflected in brain’s activity. It has been known for about fifteen years that the activity patterns in the brain allow us to identify which object a person is looking at. So, mind reading is already possible in a rudimentary form (laughs). But the "reading" of information from the brain is not the focus of our research. We want to decipher why these activity patterns look the way they do and which properties matter to our brains. To answer this question, we also want to use various characteristics such as color, shape or "has something to do with nature", "can move", "valuable" or "something with fire" as connecting elements. I believe that only by uncovering these properties we can really understand how the brain processes objects. Of course, it is not guaranteed that all properties we identify make as much sense as those we have found in our previous behavioral tasks. But, of course, this makes us all the more curious about what we are about to discover.
The interview was conducted by Verena Müller.