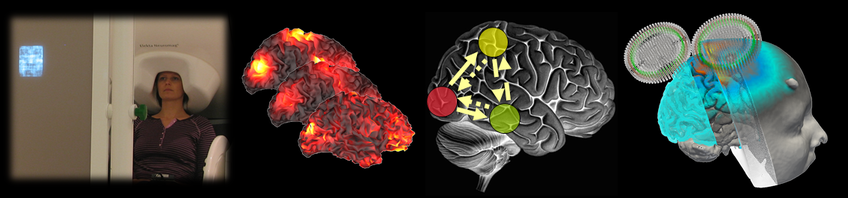
Brain Networks
The performance of the human brain is based on selection, exchange and integration of information via complex networks of neurons. The overarching goal of our working group is the development of methods to investigate, model, and understand these networks and how they give rise to human behavior and cognition. To this aim, we work on the following subjects:
Modeling of Brain Stimulation: Modelling of the biophysical and neuronal processes underlying transcranial electrical or magnetical stimulation.
Models of Neuronal Circuits: Modeling of the dynamical interaction between neuronal populations and the cognitive functions they constitute using neuronal mass models.
Source Reconstruction: Reconstruction of the electrical brain activity on the basis of EEG and MEG data via field calculation methods.
Artificial Intelligence & the Brain: Studying the rleationship between brain dynamics, cognitive function, and performance of AI.
Tractography: Investigation of the anatomical basis of the neuronal networks via MRI, in particular diffusion weighted MRI.
- OPM-Lab: Establishing an MEG lab with optically pumped magnetometers.
Cognition: Application of the above mentioned methods to research on music and language processing.
Introduction
How is it possible to explain the astonishing capabilities of the human brain on the basis of its anatomy and physiology? It has been accepted for a long time that different brain functions have different spatial representations in the brain. This segregation principle has been confirmed by numerous studies. For example, it has been shown repeatedly that certain cortical areas seem to be specialized in the visual perception of faces or houses, respectively. However, segregation alone is not sufficient to explain the complexity of brain function. We know that the psychological functions of humans are highly interdependent. For example, actions depend in a complex way on perception, attention and emotional status. Functional integration is therefore the second important functional principle of the brain. Taking a look at the anatomy of the neural system confirms this: neurons and neural populations are indeed interconnected in a very complex way. In order to investigate how the brain is able to perform, it is therefore necessary to study the anatomical connection patterns, to model the interaction between neuronal populations, and to validate these models using observations and measurements. In the following, we will describe how we might be able to achieve these goals using non-invasive methods in healthy volunteers.
Modeling of Brain Stimulation
Non-invasive brain stimulation allows influencing brain activity through externally applied electric currents or magnetic fields. This includes transcranial magnetic stimulation (TMS) and transcranial electric stimulation (TES). These methods are used for studying the functional organization of the brain as well as for therapeutic and diagnostic purposes. However, the success of the stimulation is often quite variable between experiments and subjects. This is most probably due to interindividual variability in conjunction with our ignorance of the actual mechanisms of stimulation. Therefore, our research aims at modelling the entire processing chain of non-invasive brain stimulation, starting with the induced electric fields, via the effect of these fields onto the behavior of neurons and the thereby altered dynamics of neural circuits, to the resulting physiological, clinical, and behavioral effects [27]. This will increase the predictability of experiments and treatments and significantly increase the applicability of non-invasive brain stimulation techniques in medicine and research [28-33].
Models of Neuronal Circuits
The existence of anatomical connections between particular neural populations does merely reflect the potential for information transfer, not the information transfer itself. In order to investigate, if, how, and to what degree brain areas really interact, mathematical models are used. In order to keep these models tractable and descriptive for the most important properties of the neural tissue at the same time, we use so-called neural mass and neural field models. In these models, many similar neurons are lumped together and are represented jointly by the relationship between their mean input and mean output. A similar description was developed for the transfer properties of bundles of axons [22]. An interesting feature of these models is that they can be used to predict measurements, such as electroencephalography, magnetoencephalography and functional magnetic resonance imaging [15-17]. Therefore, they are generative models and it is in principle possible to estimate their parameters, such as connectivity strengths, from measured data [15]. Based on this, one can model stimulation or behavior dependent variation of brain signals at the level of neural populations. This technique is referred to as Dynamic Causal Modeling. For example, the detection of deviants in a uniform stream of stimuli has been explained within a hierarchy of cortical neural populations [18]. Finally, neural mass models were used to explore how local cortical wiring patterns, so-called canonical microcircuits, implement basic building blocks of cognition, such as working memory, simulus gaiting, and change detection [19-21]. Importantly, most of the developed modeling techniques are made publically available in a Python based toolbox called pyRates [23] (see Software→pyRates).
Source Reconstruction
Electroencephalography (EEG) and magnetoencephalography (MEG) yield relatively direct and millisecond accurate information on the activity of nerve cells. However, the signal of each sensor represents a mixture of activity in many parts of the brain. In order to reconstruct the true activity time courses (sources) in different parts of the brain, we need to demix these signals. For this purpose, it is first necessary to quantify the influence of each source onto each sensor. The solution of this so-called forward problem requires modeling of the electric properties of the different tissues of the head. In a second step, the inverse problem needs to be solved. This problem is essentially non-unique and requires sensible assumptions on the sources or additional information from other brain imaging modalities. This fact makes source reconstruction a challenging problem, which has been subject to intensive research [24-26].
Artificial Intelligence and the Brain
In recent years, artifical intelligence has reached levels of sophistication that match, if not surpass that of humans in many areas. Beyond the classical areas of rule-based decision making (e.g., in chess playing) this now extends to core human faculties like language and verbal reasoning. This raises a number of extremely interesting questions, such as wether and to what extent natural language processing (NLP) systems work in a way that is similar or comparable to the way the human brain works. Recently, we started a research program aiming at the relationship between between language processing, biophysical whole brain modeling (based on neural masses, see above), and NLPs such as transformer models (e.g., GPT, BERT).
Tractography - the Reconstruction of the Network of Nerve Fibers
In order to reconstruct the course of nerve fibers and hence the blueprint of the neuronal circuitry, several classical methods are available. For example, the migration of tracer substances along fibers can be observed or the fiber pathways can be reconstructed using series of polarized light micrographs. However, all these methods can only be applied to dead material or in animal models. Therefore, it is difficult to directly investigate the networks underlying the specific cognitive faculties of humans. In recent years, the emergence of diffusion weighted magnetic resonance imaging offered a possibility to in vivo monitor the direction-dependent mobility of water molecules, which allows for inferences on the microstructure of the tissue, in particular on the orientation of nerve fibers. Based on a mathematical modeling technique, called tractography, it is possible to reconstruct the probable courses of nerve fiber tracts [1-7]. This methodology offers a way to image the anatomical connectivity in the living human brain. For example, we were be able to demonstrate separate brain networks for the processing of different types of grammar, investigate the development of the human language network and to explore specific connections between the neural systems dedicated to auditory and visual perception [8-10]. Moreover, one can map differences in particular tissue properties along fiber bundles [2-3], which may be related to certain diseases or cognitive capabilities. Finally, it is possible to compute for each portion of the cortex a specific connectivity profile. Under the premise that the connectivity of a brain structure with the rest of the brain is of great importance for its function, the comparison of these connectivity profiles yields information on the division of the cortex into functional units. This methodology is referred to as functio-anatomical parcellation [11-14]. A more detailed treatment of this issue is found in [13]. Hence, using tractography we are able to elucidate the anatomical aspects of both segregation (by reconstruction of parcellations) and integration (by quantifying the connectivity between brain areas). It is of paramount importance that this can be done in a non-invasive way in normal healthy human subjects, which allows us to connect this information with the specifically human faculties of the brain.
OPM Lab
Optically pumped magnetometers (OPM) are small devices capable of measuring tiny changes of magnetic fields down to the order of a single femtotesla. Such sensors use a laser to bring atoms of an alkali vapour (here rubidium) into a state of high energy. The photons of the laser can only interact with low energy atoms, i.e. the more atoms enter the high energy state the more transparent the vapor becomes for the laser. Changes of the surrounding magnetic field cause changes of this equilibrium and the transparency changes. This can be measured by a photo-sensor. Since recently, such devices are available at the market, e.g. via Quspin (quspin.com).
In magnetoencephalography, the OPM sensors may replace the commonly used SQUID sensors to some extent. At the upside, the OPMs are of relatively small size (12x17x24mm³) and they come without the need for complex cryogenics. Outside their housings, the sensors stay at about room temperature, while in the center Rubidium vapor is generating via laser heating. No weekly Helium refill as with the classical MEG device needed. At the downside, the OPMs only work at background magnetic fields which are weaker than about 50nT. They also have a much smaller bandwidth (about 150Hz) compared to the classical SQUIDs (up to 2000Hz). However, their advantages may open new avenues for the research of the human brain and mind.
Their small geometrical size makes it possible to place the OPM sensors at the surface of the volunteer's heads similarly to the electrodes in EEG measurements. Such a sensor placement reduces the distance between sensors and active brain tissue and maximizes therefore the signal-to-noise ratio of the recorded data. Furthermore, the measurement is less sensitive the head movements because the sensors move with the head. Additionally, the same set of sensors can also be arranged over other parts of the body, e.g. over the spinal cord for a completely different type of measurements.
This is all very interesting, however, there is no OPM-based magnetoencephalography device available at the market. Hence, we bought a set of such sensors and are now busy with the setup of the lab. The ultimate goal being to routinously invite volunteers and record time courses of their brain acitivity while they perform on certain cognitive tasks.
Cognition
The ultimate research goal of the group "Brain Networks" is the combination and integration of the modeling and anaylsis approaches detailed above to shed light onto the way the brain achieves its stunning functionality. So far, we have investigated how basic building blocks of cognition, such as working memory, information gating, and change detection, might be performed by the cortical circuitry [15,19-21]. Furthermore, first attempts to account for higher cognitive function have been made for speech processing [19-20] and musical pitch perception [29-30].
Literature
[1] T.R. Knösche, A. Anwander, M. Liptrot, T.B. Dyrby: Validation of tractography – comparison with manganese tracing. Human Brain Mapping 36(10):4116-34 (2015)
[2] T. Riffert, J. Schreiber, A. Anwander, T.R. Knösche: Beyond fractional anisotropy: extraction of bundle-specific structural metrics from crossing fiber models. NeuroImage 100, 176–191 (2014)
[3] J. Schreiber, T. Riffert, A. Anwander, T.R. Knösche: Plausibility Tracking: A method to evaluate anatomical connectivity and microstructural properties along fiber pathways. NeuroImage 90, 163-178 (2014)
[4] D.K. Jones, T.R. Knösche, R. Turner: White matter integrity, fiber count, and other fallacies: the do’s and don’t’s of diffusion MRI. NeuroImage 73, 239-54 (2013)
[5] R.M. Heidemann, A. Anwander, T. Feiweier, T.R. Knösche, R. Turner: k-space and q-space: Combining ultrahigh spatial and angular resolution in diffusion imaging using ZOOPPA at 7T. NeuroImage 60(2), 967-978 (2012)
[6] M. Descoteaux, R. Deriche, T.R. Knösche, A. Anwander: Deterministic and probabilistic tractography based on complex fiber orientation distributions, IEEE Transactions on Medical Imaging 28, 269-286 (2009)
[7] E. Kaden, T.R. Knösche, A. Anwander: Parametric spherical deconvolution: Inferring anatomical connectivity using diffusion MR imaging, NeuroImage 37, 474-488 (2007)
[8] A.D. Friederici, J. Bahlmann, S. Heim, R.I. Schubotz, A. Anwander: The brain differentiates human and non-human grammars: Functional localization and structural connectivity. Proceedings of the National Academy of Sciences of the United States of America 103(7), 2458-2463 (2006)
[9] J. Brauer, A. Anwander, A. D. Friederici: Neuroanatomical prerequisites for language functions in the maturing brain. Cerebral Cortex 21(2), 459-466 (2011)
[10] H. Blank, A. Anwander, K. von Kriegstein, K. (2011). Direct structural connections between voice and face-recognition areas. The Journal of Neuroscience 31(36), 12906-12915 (2011)
[11] D. Moreno-Dominguez, A. Anwander, T.R. Knösche: A Hierarchical Method for Whole-Brain Connectivity-Based Parcellation. Human Brain Mapping 35, 5000–5025 (2014)
[12] M. Ruschel, T.R. Knösche, A.D. Friederici, R. Turner, S. Geyer, A. Anwander: Connectivity architecture and subdivision of the human inferior parietal cortex revealed by diffusion MRI. Cerebral Cortex 24(9): 2436-2448 (2014)
[13] T.R. Knösche and M. Tittgemeyer: The role of long-lange connectivity for the characterization of the functional-anatomical organization of the cortex, Frontiers in System Neuroscience 5:58. (Epub 2011)
[14] A. Anwander, M. Tittgemeyer, A.D. Friederici, D.Y. von Cramon, T.R. Knösche: Connectivity-based cortex parcellation of Broca’s area, Cerebral Cortex 17(4), 816-825 (2007)
[15] P. Wang, T.R. Knösche: A realistic neural mass model of the cortex with laminar-specific connections and synaptic plasticity – evaluation with auditory habituation, PLoS ONE 8(10) e77876 (2013)
[16] A. Spiegler, T.R. Knösche, K. Schwab, J. Haueisen, F.M. Atay: Modeling brain resonance phenomena using a neural mass model, PLoS Computational Biology 7(12) (2011)
[17] A. Spiegler, S. Kiebel, F. Atay, T.R. Knösche: Bifurcation analysis of neural mass models: impact of extrinsic inputs and dendritic time constants. NeuroImage 52(3), 1041-1058 (2010)
[18] M.I. Garrido, K.J. Friston, S.J. Kiebel, K.E. Stephan, T. Baldeweg, J.M. Kilner: The functional anatomy of the MMN: A DCM study of the roving paradigm. NeuroImage 42 (2), 936-944 (2008)
[20] T. Kunze, J. Haueisen, T.R. Knösche: Emergence of Cognitive Priming and Structure-building from the Hierarchical Interaction of Canonical Microcircuit Models, Biological Cybernetics 113(3): 273-291 (2019)
[21] S.C. Chien, B. Maess, T.R. Knösche: A generic deviance detection principle for cortical On/Off responses, omission response, and mismatch negativity, Biological Cybernetics (2019)
[22] H. Schmidt, T.R. Knösche: Action Potential Propagation and Synchronisation in Myelinated Axons, PLoS Computational Biology, 15(10): e1007004 (2019)
[23] R. Gast, D. Rose, H.E. Möller, N. Weiskopf, T.R. Knösche: PyRates: A Python framework for rate-based neural simulations. PLoS One, 14(12):e0225900 (2019)
[24] J. Vorwerk, J.H. Cho, S. Rampp, H. Hamer, T.R. Knösche, C.H. Wolters: A guideline for head volume conductor modeling in EEG and MEG. NeuroImage 100, 590-607 (2014)
[25] M. Fukushima, O. Yamashita, T.R. Knösche, M. Sato: MEG source reconstruction based on identification of directed source interactions on whole-brain anatomical networks. NeuroImage 105, 408–427 (2015)
[26] J.H. Cho, J. Vorwerk, C.H. Wolters, T.R. Knösche: Influence of the head model on EEG and MEG source connectivity analysis. NeuroImage 110, 60-77 (2015)
[27] G.B. Saturnino, A. Thielscher, K.H. Madsen, T.R. Knösche, K. Weise: A principled approach to conductivity uncertainty analysis in electric field calculations. NeuroImage 188, 821-834 (2019)
[28] K. Weise, Ol. Numssen, A. Thielscher, G. Hartwigsen, T.R. Knösche: A novel approach to localize cortical TMS effects. NeuroImage (in press)
[29] S.G. Kim, T.R. Knösche: Intracortical myelination in musicians with absolute pitch: quantitative morphometry using 7-T MRI. Human Brain Mapping 37, 3486–3501 (2016).
[30] S.G. Kim, T.R. Knösche: Intracortical myelination in musicians with absolute pitch: quantitative morphometry using 7-T MRI. Human Brain Mapping 37, 3486–3501 (2016).
[31] O. Numssen, A. Thielscher, G. Hartwigsen, T.R. Knösche, K. Weise: Efficient high-resolution TMS mapping of the human motor cortex by nonlinear regression. NeuroImage 245: 118654 (2021)
[32] K. Weise, O. Numssen, B. Kalloch, A. Zier, A. Thielscher, G. Hartwigsen, T.R. Knösche: Precise motor mapping with transcranial magnetic stimulation. Nature Protocols 18, 293–318 (2022)
[33] K. Weise, T. Worbs, B. Kalloch, V.H. Souza, A.T. Jaquier, W. Van Geit, A. Thielscher, T.R. Knösche: Directional Sensitivity of Cortical Neurons Towards TMS Induced Electric Fields. Imaging Neuroscience, in press (2023)