"Understanding general organizational principles to comprehend complex structures like the brain"
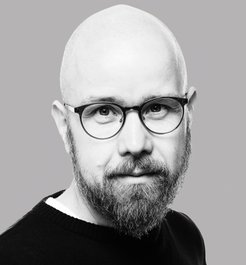
Your research is about "neural data science". What exactly does that mean?
We want to develop methods with which complex data can be evaluated and patterns recognized in it. Data are like a kind of landscape in these high-dimensional spaces. So far, I have worked a lot with single-cell analyses. In embryonic development, for example, you can observe how the cells divide and move and what patterns emerge. If you sequence these cells at the same time, i.e. their genes, you can see that they also have different genetic configurations. Now it would be interesting to know, for example, how the two are connected, how one affects the other. And yes, there really do seem to be certain developmental paths that the cells take in both perspectives. For example, a stem cell has a certain genetic profile and develops from that in different directions and then differentiates into specific cell types. And sometimes, unfortunately, cancer can develop as a result of a "wrong" path.
Such self-organizing structures and processes can of course also be recognized in the brain - and also corresponding deviations from them, for example Alzheimer's disease. We want to contribute to the machine detection of such patterns and possible irregularities, which can also lead to diseases. No one can manually look at the data that can be recorded on a patient anymore. Automated evaluation procedures are needed for this.
And I am particularly interested in how such complex data can be visualized in the first place. In other words, how to process it in such a way that it can be explored further. When the measurements become more and more complex, it also becomes more and more difficult to evaluate the data. Simple statistics are often not enough. My vision is to be able to generate maps of complex data, which the scientist or doctor can then use to better understand the correlations.
Which of your projects have had a particular impact on you so far?
I have always enjoyed visualizing data the most. In our paper in Nature Communication, for example, we illustrated how the cells in a zebrafish organize themselves during embryonic development. And how the different cell types then arrange themselves along the embryonic body axis and thus ultimately determine the blueprint for the organs. It was a matter of processing this new kind of data in such a way that one can continue to work with it and answer biological questions. In doing so, we again used methods that were developed here at the Institute to make nerve pathways in the brain more visible. With these methods, we were then able to visualize very well the common patterns in the movement of about 10,000 cells.
I also particularly liked a project with Jan Huisken in which we thought about how "intelligent microscopes" should be constructed so that complex systems can be explored across different scales. To do this, the microscope should not blindly record everything, but "learn" with each measurement, for example through a statistical model. The next time, it would then have to measure where we still know too little. This would result in much smaller amounts of data and much more precise measurements. Especially with living cells, many microscopes have so far often measured artefacts because the cells have not always survived the measurement unscathed. The idea of cross-scale imaging is of course also very interesting for neuroanatomy. In my time as a postdoc here in the Neurophysics department at MPI CBS, I was involved in a study together with the Paul Flechsig Institute, where we put out some initial ideas in a NeuroImage paper about how to bring microscopy and MRI together.
You have done a lot of work with zebrafish embryonic cells. What do they have to do with neurons in the brain?
(laughs). I would like to leave that to the cell biologists. What I can say is, even, or especially, in simple organisms, it is easy to observe how individual cells interact with each other. Cells can "perceive" their environment and also exchange information with each other, for example via messenger substances and receptors, and in this way ultimately also manage to build a complete embryo. Understanding such general organizational principles on a simple level is fundamental to understanding at some point how complex organisms or structures, such as the brain, organize themselves in nature. To date, we do not know why a large number of neurons can suddenly think or when exactly this happens. Understanding such self-organization from the simple to the complex is actually something I am very interested in. I am sure that there are general basic rules of self-organization in nature that occur again and again.
In what way?
Even relatively simple things can interact with each other. Let's take a single ant. It may not be very smart in itself (like a single cell). But it can communicate with the other members of its species. The whole ant colony is then basically the actual organism that functions and can solve quite complex tasks. Some of what Deborah Gordon, one of the leading ant researchers, has found about the organizational mechanisms of ants can probably also be applied to cells - and possibly also to complex systems such as neuronal networks. For example, she had shown that when ants have to decide which route to take in order to find food, they use the same "tactics" as we do when we want to use our computer networks efficiently. In the future, I also want to investigate methods to simulate these basic principles. For example, how much communication does it take for the overall construct to work reliably?
Besides this research, the main goal of your new group is to support other scientists at the Institute in their work. How do you envisage this?
Exactly, we want to help other scientists in dealing with their data, from classical statistics to machine learning. We are interested in the whole range of data that play a role here at the Institute, such as MRI images, EEG data or genetic data, and also in the most diverse questions that lie behind them. I am very curious to see what emerges. Each type of measurement looks at the person from a different perspective, depending on whether you take a blood sample or a brain scan. Ultimately, of course, we would like to know how we can bring the measurements from the different "perspectives" together and whether we can find certain patterns here that ultimately help us to better understand the brain and its function.
And we also want to offer various courses, for example on Python or artificial intelligence, or more specific topics such as probabilistic programming, a method with which you can build relatively complex statistical models and then analyze them using measured data.
We look forward to it. Thank you very much for the interview.