Biomechanical Modeling of Cortical Layers
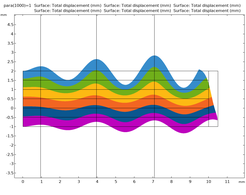
Simulation of the deformation of six neo-Hookean hyperelastic layers, where each color corresponds to a different layer starting from first layer shown in blue to the last layer in violet. The thicknesses vary with curvature. The grid is the material setup before deformation, the vertical lines indicate the position at which the layer thicknesses are measured after deformation. For simulation, a function was used on top as well as on the bottom boundaries, where the amplitude of the bottom function was reduced to 60%, in order to get a different curvature when compared to the top. After deformation the general behavior agrees with Bok’s observation, i.e. at high curvature the thickness is larger when compared to a neighboring section with less curvature.
The spatial resolution improvements in structural MRI brings us closer to image structural and functional aspects at the intracortical level in-vivo. However, the current resolution of MR imaging cannot faithfully resolve the geometry of individual cortical layers. There is a variety of methods to estimate layers thickness from the extracted cortical geometry but they are either based on computationally convenient yet biologically implausible assumptions such as the Laplace or equidistant model, or purely observational lacking a solid biophysical foundation. The most reliable method used to estimate the location and size of cortical laminae so far, is a geometrical model the so called equi-volume model (based on Bok’s histological observations). However, with a purely observational model we cannot quantify the influence of varying tissue properties e.g. elasticity across the cortex and its potential influence on local geometry of intracortical layers. Therefore, we propose a framework which is composed of a hyperelastic biomechanical model, the so called neo-Hookean hyperelastic material model, for simulation of intracortical layering with the aim of extending and improving the existing models.
Our simulations will be carried out to verify tissue parameter dependency on brain region and local cortex geometry. To evaluate our simulation results, we will use ex-vivo MR images of primary visual cortex. We will further use simulation results to develop a regression model for layer thickness depending on estimated material properties and local 2D/3D curvatures for direct access to layering in real MRI data. Taking into account the influence of tissue properties on the geometry of intracortical layers, we can better adapt our structural and functional measurements to different regions of the brain with varying curvature and tissue properties related to the changes in mechanical properties caused by aging or disease.