Reducing the level of artifacts in quantitative parametric maps at 3T and 7T
Multi-parametric quantitative MRI measures different MR parameters and offers the potential to characterize human brain microstructure. An efficient implementation of this concept, the multi-parameter mapping (MPM) protocol, uses 3 differently weighted multi-echo 3D-FLASH volumes to simultaneously quantify R1, R2*, proton density (PD) and magnetization transfer (MT) (Weiskopf et al., 2013, Front Neurosci, 7, 95). However, MRI artifacts are propagated into the quantitative maps and obfuscate meaningful physical values. To improve the reliability of the generated MPMs, rigid head motion and B0-fluctuation were measured during acquisition at 7T and corrected in a prospective or post-processing approach.
Optical prospective motion correction (Kineticor, HI) was used to track head movement. It has been previously shown to reduce the level of motion related artifacts in the parametric maps (Callaghan et al., 2015, Front Neurosci, 9, 97). By monitoring the magnetic field fluctuations with free induction decay (FID) navigators and performing phase correction during image reconstruction, dynamic field changes due respiration were addressed, effectively decreasing the level of regional patchiness and blurring. The figure below shows artifact reduction in R1 maps due to navigator correction (bottom row) and optical prospective motion correction (PMC, right column). Dynamic B0-fluctuation correction using FID navigators results in increased R1 homogeneity in white matter by reducing patchy hyper-/hypo-intensities (red arrows). Blurring of the gray-white matter boundaries (yellow arrows) caused by motion is reduced in motion-corrected maps.

To correct artifacts of unknown origin, we employed general function approximators in the form of CARE-Net/U-Net like feed forward neural networks (Weigert et al., 2018, Nat Methods, 15, 1090–1097 / Ronneberger et al., 2015, MICCAI 2015, 234–241). One specific example are ringing artifacts of unknown origin, appearing in maps acquired at 3T (a). Training labels were generated by averaging multiple acquisitions showing the ripples in different locations, which yielded mostly artifact free images. The trained model was used to correct the weighted multi-echo images before MPM computation. Corrected images showed reduced artifact, while changes to unaffected regions of the image were minimized (b). The figure below shows exemplary slice of corrupted T1 weighted input image (first echo) with ringing artifacts in the frontal areas (a), corrected output from neural network (b) and variance map showing model intrinsic uncertainty of the corrected image (c). This approach preserves image features considerably better than other options to address the specific artifact like Gaussian blurring or removing k-space lines.
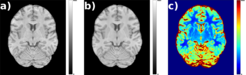
Using these different methods we are able to substantially reduce the severity of the most pronounced artifacts in the quantitative maps. We showed qualitative improvement of parametric maps by reducing the blurring and ringing coming from the rigid head motion. B0-fluctuation correction resulted in the decrease in variance of dorsal white matter voxels by ~5% (in R1, R2*, PD map). The neural network was able to reduce the artifact in a test dataset by 12 dB while preserving artifact free image areas (multi scale structural similarity (MS-SSIM) in artifact free ROI over all contrasts 0.89).