Evaluating different k-space undersampling schemes with iterative and deep learning image reconstruction for fast multi-parameter mapping (MPM)
The well-established approaches to speeding up microstructural imaging using quantitative multi-parameter mapping (MPM, (Vaculčiaková et al., 2022)) rely on Cartesian undersampling. Image reconstruction of the underlying multi-echo gradient echo (ME-GRE) data is performed echo by echo. This study explored different k-space undersampling schemes, i.e., elliptical Poisson disc compressed sensing (CS) and CAIPIRINHA, in comparison to equispaced Cartesian. The performance of iterative image reconstruction (ENLIVE, (Holme et al., 2019)) and machine learning based Deepcomplex-MRI (DCMRI, (Wang et al., 2020)) on these datasets was evaluated.
Fully sampled, 1mm isotropic resolution MPM datasets (a pair of PD- and T1-weighted 3D ME-GRE acquisitions with 8 equidistant echoes) were acquired on eleven healthy volunteers employing prospective motion correction at 3T. Fourier transform was applied in the readout direction, resulting in a stack of 2D k-space planes/slices. The resulting 2D k-space planes for each volume were processed separately, retrospectively undersampled and fed into the different reconstruction algorithms using all 8 echoes stacked together. The dataset of one volunteer was held back for evaluation. Results were compared against root sum of squares, coil-combined, fully-sampled reference data in terms of peak signal-to-noise ratio (PSNR), structural similarity metrics (SSIM), and qualitative appearance.
ENLIVE was applied using default parameters with 12 iterations in about 40 minutes per volume on 128 compute cores. DCMRI was adapted to process all 8 echoes simultaneously by introducing an additional input/output dimension and modifying the necessary data preparation steps. The residual blocks were adjusted to use convolutions with 64 output channels for the hidden states. The training was performed separately for each sampling pattern employing an accelerated High-Performance-Computing node with 4x NVIDIA A100 GPU (40 GB HBM2 memory) for about 12 hours each.
After training, inference for each volume was performed in less than 4 minutes on a single GPU. In general, image quality was rather high even at high 9x acceleration factors (see figure). Average PSNR and SSIM were comparable between k-space schemes and reconstruction methods (see figure). A steeper decrease with higher acceleration factors was noticeable for DCMRI. One potential explanation is little robustness of the DCMRI architecture to very noisy data that might be handled by introducing batch normalisation or dropout layers and potentially focusing stronger on image foregrounds during training. Independent of the method, a similar quality reduction was found for longer echo times (see figure). Visual inspection shows that Cartesian and CAIPIRINHA sampling schemes resulted in more structured artifacts specifically when using DCMRI reconstruction (see figure). ENLIVE tends to enhance noise and shift the average image intensity for slices with little signal, leading to visible stripe-like structures at the top and bottom in the coronal and sagittal views (see figure).
We compared state-of-the-art ENLIVE to multi-echo DCMRI, which showed high image quality even at 9x acceleration. The much shorter reconstruction time of DCMRI promises to facilitate routine high throughput applications and online visual image inspection. Future work will concentrate on establishing advantages of joint multi- echo DCMRI and ENLIVE reconstruction for calculation of MPMs and applying DCMRI to high-resolution data that suffers from long reconstruction runtimes.
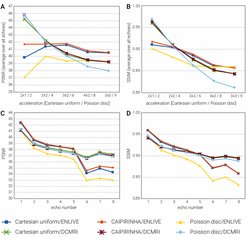
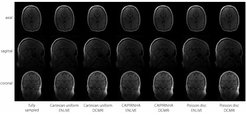
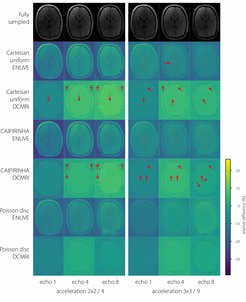